Pop quiz: What is big data?
Actually, don’t answer that. Everyone can easily recite the same quote: “It’s the process of analysing huge amounts of data to draw actionable insights that are beneficial to your business.”
In practice, this can be a difficult area to navigate. Big data covers an immensely wide area, and different datasets can yield different results; knowing what to use when and for what purpose can soon turn infinitely confusing.
In the banking industry, where data is religiously collected and archived, it seems like the perfect playground to run big data projects. However, the key to a successful big data project is when it can make a positive impact on your bottom line. There is no use for data collection if it’s not being used effectively.
Since early 2010s, Singapore banks have kicked off what is today a whole new discipline in banking operations by taking advantage of big data analytics. Within the span of a decade, Singapore banks have gone beyond just rudimentary proof-of-concept projects, to recording positive results in operation efficiency as well as customer satisfaction.
Client Processes
With the help of machine learning, big data can help automate manual processes that are currently used for corporate and institutional client onboarding, such as client due diligence. It can also help speed up SME lending processes, as bank statements provided by clients can be processed and financial calculations can be performed automatically, saving hundreds of hours a week.
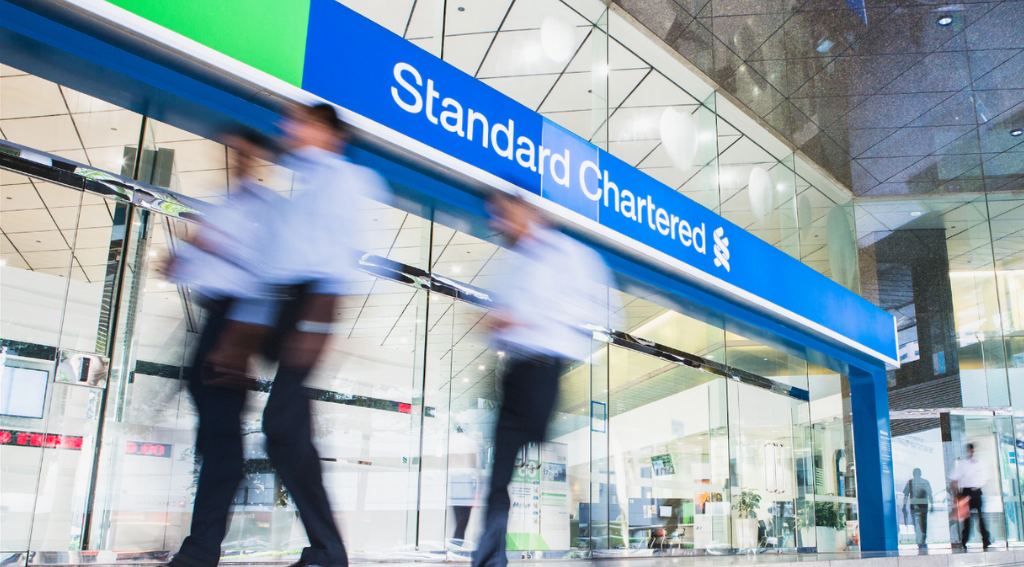
Source: Standard Chartered
Standard Chartered Bank is currently working with startup Instabase to automate due diligence processes used for business criteria, sourcing of information from various external public and private registries on sanctions and adverse media mentions matched against various watch lists.
In a 2017 interview, Citibank has also noted that they are using big data to automate reporting to regulators, eliminating manual work and errors. Additionally, the bank is also using big data to help in the decision making process for growing or winding down specific portfolios.
HR operations
Data analytics aren’t only useful to analyse customer data, but also to streamline internal operations. For example in recruitment, data analytics tools are used to assess job applicants’ profiles, significantly cutting the number of hours recruitment officers spend on the initial screening of applicants.
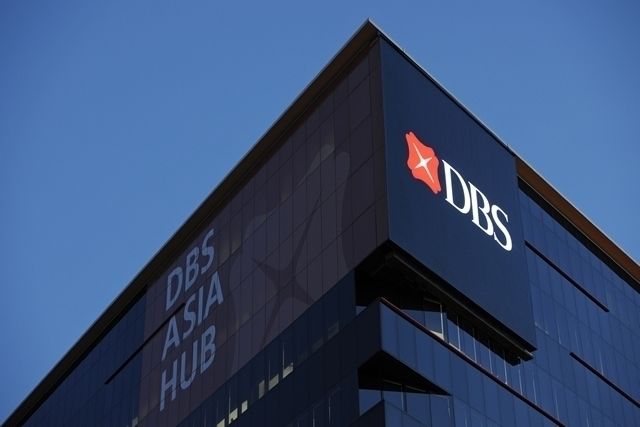
Source: DBS
As early as 2011, Singapore’s DBS Bank did an overhaul of its HR operations, using big data analytics to boost recruitment staff productivity and reduce attrition rates. The bank set up a Human Capital Analytics team within the department to evaluate trends and determine risk factors for early employee attrition. Since then, the bank has recorded a lowered attrition rate of 18 percent compared to the previous 27 percent.
Similarly, OCBC Bank has also been noted to have used big data since 2015 to customise training and hiring programs.
Responding to cyber incidents
With so much information being exchanged across the banking network, it is important for banks to secure not only the network but also its data centres. Big data can analyse threat patterns and identify any suspicious activities that could compromise a bank’s internal system.
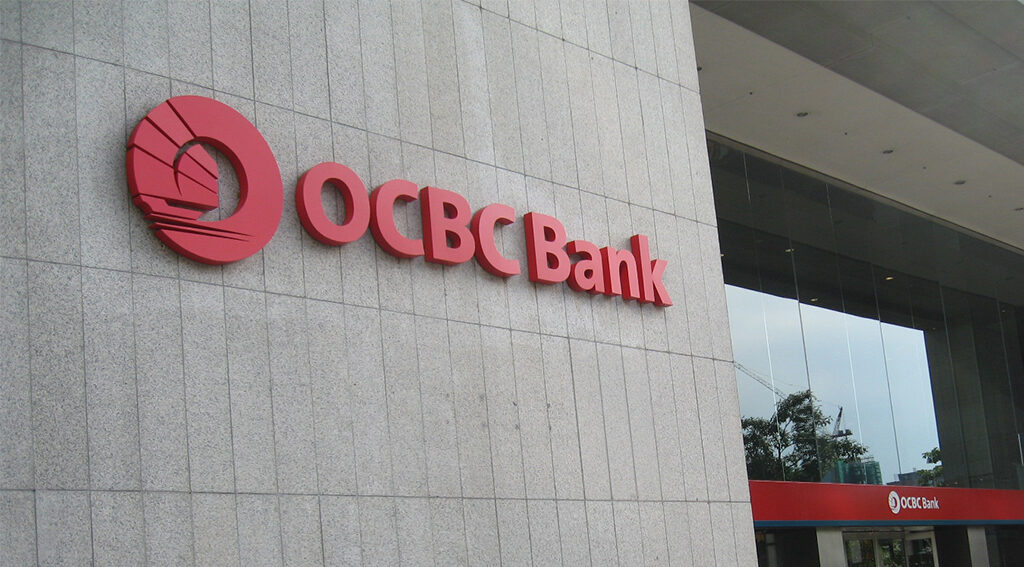
Source: OCBC Bank via Wikimedia Commons
Since 2017, OCBC Bank has used predictive analytics and big data to prevent 55 percent of cyber threats from materialising, as well as resolve 62 percent of issues encountered right at the onset. This coupled with the use of voice assistants to automate tasks such as information retrieval and call activation during crises, has helped free up manpower for key decision-making during incidents.
Auditing bank branches and ATM network
Identifying the risks of a bank branch is tedious if not near impossible if done manually. Big data is used to identify any potential problems in any particular branch, which might require an audit. It can also help identify customer needs more accurately, using data such as customer flows, ATM load, and call centre volumes. This allows the bank to develop better products and services, thus reducing the level of complaint.
Image Credit: Wikimedia Commons
One of the earlier projects that DBS bank embarked on was in fact to improve the bank’s ATM services by figuring out the sequence in which ATMs should be filled. With data analytics, the bank was able to understand the patterns of ATM cash flow and to predict when ATMs would run out of cash (also known as a cash out).
Since implementing predictive analytics, the bank has recorded a 98 percent reduction in cash out, as well as 92 percent reduction in customer complains. The bank’s data noted that in a year, their customers saved 800,000 hours worth of ATM queue time.
Anti money laundering
Traditionally, bank processes for anti money laundering is reliant on a rule based approach. In other words, the system flags any transaction that violates a set of rules. A human will have to then manually sift through and review the transaction to identify any suspicious activity.
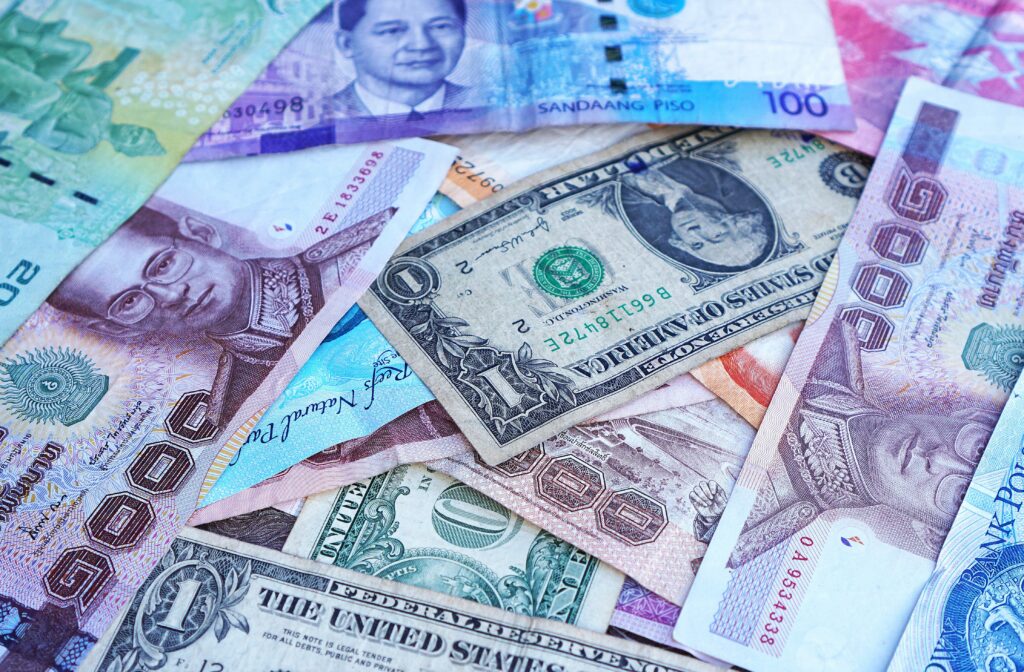
Source: Image via Pexels
Data analytics can automate that process, significantly reducing human workload while improving accuracy. In a report by the Association of Banks in Singapore (ABS), an unidentified member bank reported a 50-60 percent reduction in false positives in the name screening module, while the transaction monitoring module resulted in a 40 percent reduction in false positives. Additionally, it was able to detect new suspicious patterns which resulted in a 5% increase in true positives.
OCBC Bank also reported a 35 percent decrease in the number of cases needed for human review after implementing big data analytics, as we’ve reported back in 2017. In the case of DBS, it is using big data to help detect fraud anomalies in trade finance through transactional trends instead of relying on checks on singular transactions.